In a world where artificial intelligence (AI) can beat world champions at complex strategy games, drive cars through city streets, and predict the three-dimensional shape of biomolecules, we know it can also power a transformative and long-overdue nature data revolution.
At Pivotal, we’re building AI models that help process biodiversity data at huge scale, validated by human experts, making it possible to reliably track change in the state of nature, no matter the ecosystem. But we also know that biodiversity poses a unique and complex challenge that’s beyond both the training and the application of current AI models.
Why is measuring biodiversity such a challenge?
Nature is beautifully complex and multi-dimensional, making it fiendishly difficult to track. The term ‘biodiversity’ covers everything from the microbes, plants and wildlife around us, to the interconnected systems (ecosystems) that they form with each other and their environments.
Decades of under-investment, coupled with the complexity and variety of ecosystems, has led to a global shortage of reliable data amid an intensifying biodiversity crisis. Less than 7% of Earth’s land surface has been systematically surveyed for its biodiversity, and an even smaller percentage is consistently monitored over time to quantify changes in ecosystem health. There has never been an affordable or scalable way to monitor ecosystems to produce science-based, auditable insights on what changes are really happening.
Thankfully, things are now changing as technology starts to lean into the challenge. There are now various digital tools that can capture huge quantities of biodiversity data in quick, repeatable, auditable ways – for example, acoustic recorders, different types of cameras, drones, and eDNA sampling.
Less than 7% of Earth’s land surface has ever been systematically measured for its biodiversity, and an even smaller percentage is consistently monitored over time to quantify changes in ecosystem health.
But amid the excitement around these new tools, it’s important to remember that there is no single tool that can do it all. For example, you can’t use acoustics to collect data on plants, or eDNA to track changes in species abundances. Tracking changes in the state of nature (or ecosystem health) depends on holistic measurement across the different dimensions of biodiversity – habitats, different species groups, and so on.
Measuring accurately across this wide and varying range of biodiversity dimensions requires a diverse toolbox of data technologies. The trick is to deploy a suite of tools – each of which is the right tool for the right job – and then to analyse all the data in aggregate, from all these different sources.
And the challenge doesn’t end there. While technology is revolutionising biodiversity data collection, the data must be analysed before it can be useful, which is a monumental task. Data analysis in this context means identifying species in audio files, images, and DNA samples, identifying habitat features in drone or satellite imagery, and then putting it all together to generate insights that tell us how the state of nature is changing.
At Pivotal, our core focus is to innovate the analysis of digital biodiversity data from multiple sources, to generate intuitive, meaningful metrics on how the state of nature is changing over time. Our data pipelines need to be able to deal with a variety of different data types, from ecosystems all over the world, and to use them to identify species and habitat features.
We’re developing innovative AI tools to help us take on this mammoth task, but this means we need to understand and mitigate the limitations that arise when AI is used to analyse biodiversity data.
The Limitations of Conventional AI in Ecology
We can all agree that AI has become something of an overused buzzword, often now replacing terms like ‘smart’ or ‘digital’. In many cases, the phrase “we are using AI” roughly translates to “we’ve incorporated ChatGPT”, or in the biodiversity world – “we use BirdNet in the backend”.
While there’s an abundance of useful, open-source models for species classification or detection (e.g., BirdNet, PlantNet, MegaDetector), they can’t be relied upon as the fundamental component of a system that needs to accurately track species-level changes over time. These models, designed primarily for consumer apps, are often black boxes with limited-to-no explainability. As many businesses now have financial, legal or regulatory requirements tied to understanding the state of nature, we must be able to trace species detections to reputable and accountable sources.
Another significant issue with commonly deployed, open-source classification models (such as BirdNet) is their bias towards more common species and those found in the northern hemisphere, particularly in Europe and North America. Much of the world’s biodiversity inhabits equatorial regions and areas where collecting training data is more challenging – open-source AI models often do poorly here (Figure 1). In most ecosystems, a small number of species are dominant (‘common’), comprising the majority of individuals, while the majority of species are present in much smaller numbers, and are considered locally rare (Figure 2). Open-source models tend to be much better trained on the common species, and can be poor at classifying those that occur in smaller local numbers. Such issues present a substantial obstacle to using these models. If your goal is to be able to track real change in the state of nature, your analysis pipeline must be able to handle rare species and must be globally operable. Particularly because locally rare species are often the most important for maintaining ecosystem functionality and resilience.
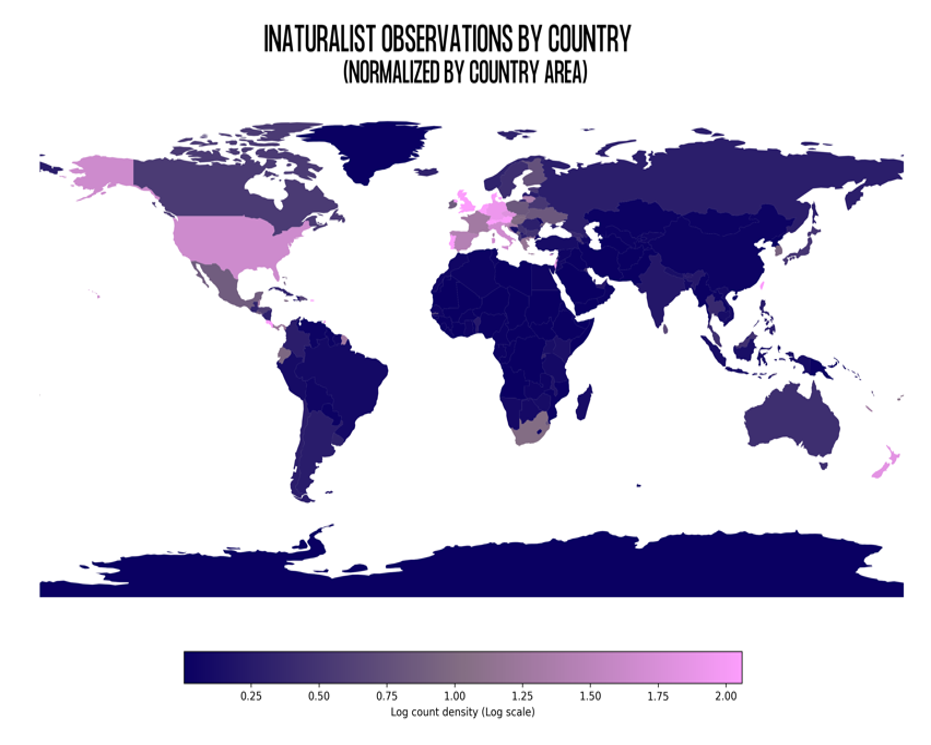
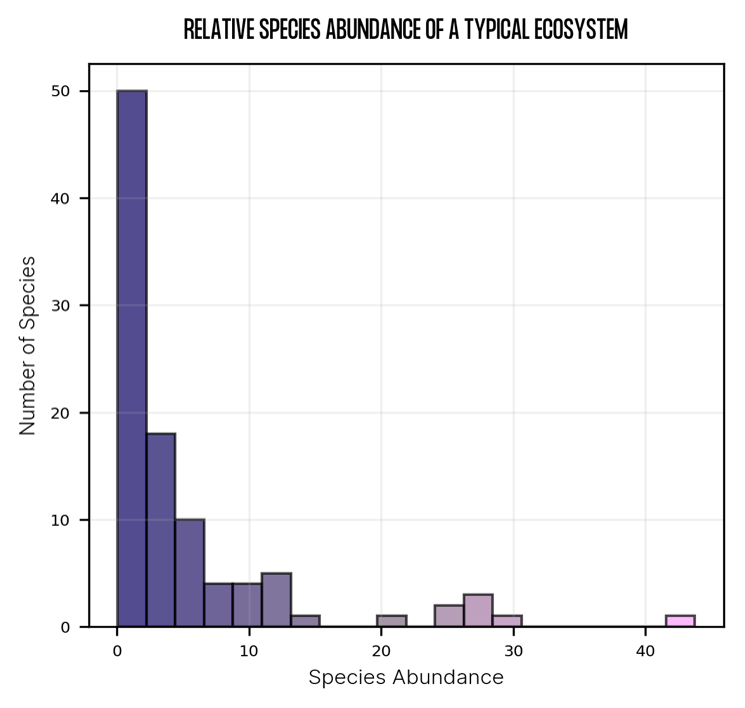
Would you trust an AI system to manage your financial data, without double checking? Would you accept the diagnosis of an AI doctor without a human medical professional’s supervision? We believe the same is true of biodiversity data, where AI models should be assisting, not driving the process. We can’t know when a model will make a bad prediction or hallucinate a species that wasn’t in fact there. So, if AI models are being used to feed directly into biodiversity calculations, we must always ask the question:
How do you assess whether changes in biodiversity metrics reflect actual ecosystem changes, or merely differences in AI model performance on certain species or in certain areas?
Our answer: Clustering and Local Models
To address these challenges, Pivotal has developed an innovative approach that combines the scalability of AI with the expertise of taxonomists: The Pivotal “CaLM” Approach, which stands for Clustering and Local Models. This innovative pipeline addresses the limitations of pure AI, while leveraging its strengths. By combining AI’s efficiency with human expert knowledge, we’ve created an auditable system that can generate highly accurate biodiversity data at massive scale.
Rather than following the more obvious approach of leaning heavily on AI prediction and using taxonomic experts merely for ground-truthing (the ‘human in the loop’ method), we’ve chosen a process that is far more auditable and just as scalable. Our AI tools act as a force multiplier for our taxonomic expert network, dramatically increasing their efficiency and reducing their annotation load.
Pivotal’s CaLM approach combines deep-learning based clustering models with location-specific classification models. These processes achieve two key things: (i) they vastly lighten our annotation burden, while retaining multiple levels of quality control by taxonomic experts; and (ii) they focus our expert annotations on the rarer and harder to classify species, ensuring these are never ignored. This is the opposite of systems that lead with AI classification, which perform better on common and easy-to-classify species, leaving many rare species (which may not have been contained in the AI training data) unaccounted for in the results, and can hallucinate species detections that are not caught by human experts. These issues get substantially worse when monitoring in more data-deficient regions of the world.
Our CaLM approach satisfies our two crucial requirements: scalability and auditability. All species identifications can be traced back to multiple taxonomic experts, and the data itself can be traced all the way back, via the AI pipeline, to the original raw data file with the precise time and location where it was collected. This ensures accountability, and our leveraging of AI allows us to rapidly scale this process across the globe, without exhausting our network of taxonomic experts. No species is detected solely by an AI model, but many detections are assigned classifications based on an expert annotation of a similar detection.
Pivotal’s CaLM approach also enables adaptability and input invariance. The same process that we use to identify species of bats from ultrasonic recordings can also be used to identify species of tree from drone fly-over videos. The building blocks might be different, i.e. using specific bat call detection and feature-extraction models, but the overall structure is the same. This gives us the unique potential to bring new sources of biodiversity data online with relatively little development time.
Future Directions in AI-Assisted Ecology
Pivotal is a company full of technically gifted, nature-loving individuals. The dual purpose of pushing technical boundaries and enabling the protection of our planet has enabled us to achieve an enormous amount of progress over the last couple of years. Assisted by some of the smartest minds in statistical ecology, our machine learning team has been constantly researching and developing new AI tools to improve upon the “CaLM” approach and push AI-assisted ecological data in new and exciting directions. Our mission is to continue to push for higher-quality, holistic insights into the state of nature, optimising the output of our world-class network of taxonomic talent and fully applying this methodology to as many species groups as we can.
If you’re interested in pushing the boundaries of AI for ecology, leading to breakthroughs in our understanding and monitoring of global biodiversity, join us! We’re always recruiting for incredible talent so please get in touch or connect with us on LinkedIn.